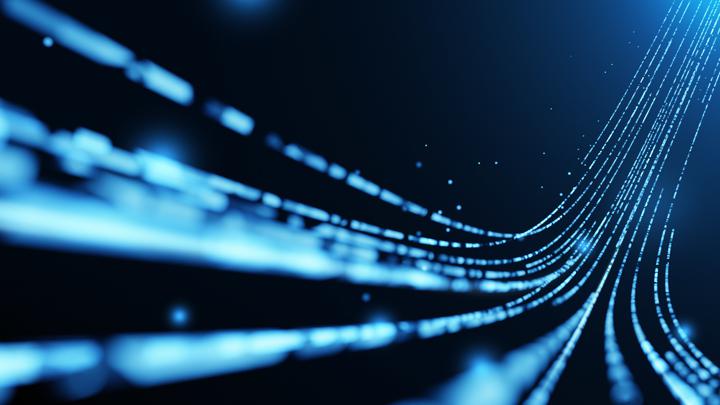
“In God we trust. Everyone else bring data.”
This quote by W. Edwards Deming aptly summarizes the significance of data for the modern enterprise.
Data: An Organization’s Most Precious Resource
Consider this: successes of companies like Lyft and Airbnb, which have brought disruptive changes in their industries, have been based on the depth of their understanding and usage of data analytics. Organizations like Vodafone and Comcast are using complex data models and building AI capabilities. Cutting-edge companies are using robots on their warehouse floors, using bots to define product pricing dynamically, and aerospace operators are using data-based insights from inflight information to develop their predictive maintenance capabilities.
Clearly, data is the foundation of today’s most successful enterprises.
As the amount of data generated by modern enterprises increases, the practices around storage, management, and analysis of this data also needs to improve. When data is so critical to operational success, any downtime in data accessibility or retrieval could result in significant losses in efficiency. In addition, business data today is so precious, that not analyzing the data to derive insights could be termed akin to hara-kiri.
Ironically, according to a report by Forrester, more than 60 percent of data in the average organization is not being analyzed.
So, what exactly are the organizations that don’t analyze most of their data losing out on?
Making Business Improvements Using Data Analytics
There is no doubt that every area of business can potentially be improved by methodically analyzing business data, understanding its implications and deriving data-based insights from it. When used smartly, data can help a business make improvements in all processes – from sales and operations to IT. Data analytics can help businesses make informed changes to counter any challenges they are facing by understanding what works and what doesn’t in their specific scenarios. Thus, in today’s world, organizations that are not making business decisions based on data may be simply groping in the dark.
According to research from PwC, data-driven organizations are 3x more likely to report significant improvement in decision-making.
Let’s look at some of the key challenges that data analytics has helped de-risking in different industry segments:
Manufacturing: Servitization is an emerging trend in the manufacturing industry. Product companies are now leveraging vast amounts of customer-centric data to offer value-added service contracts along with their product thanks to actionable, data-derived insights.
Mining: Similarly, in the mining industry, there is a ubiquitous amount of data collected, making it mission-critical for the organizations to analyze, visualize and prioritize this data in order to minimize equipment downtime and keep operations efficient and effective.
Retail: Retail enterprises are also now deep-diving into customer data to streamline their supply chain, optimize inventory, make operations more efficient and even devise new winning strategies to keep customers happy and further expand their customer base.
Technology: With exponential advances in computing techniques, the adoption of smart apps, bots, and AI is on the rise, fast becoming the pillars of modern enterprises – all of which are based on deep data analytics. Satya Nadella has aptly termed AI as the new “runtime” of the firm. In other words, AI-driven analytics is quickly becoming the norm. Organizations can now use AI/ML techniques to identify patterns in data and extract valuable insights to gain an edge over competitors.
Marketing: The customer’s needs and wants are rapidly changing in today’s world. And only the products and services that adapt quickly to meet these ever-evolving needs can survive. The new-age customer wants products and services that are personalized to his or her own specifications. In fact, today’s customer demands this personalization. Mass personalization is only feasible with the use of smart data analytics. Data captured across the customer journey needs to be collected and analyzed so that the insights derived can be used to improve the customer’s experience. By identifying meaningful patterns in customer data, smart marketers can target customers more effectively and maximize the impact of their marketing.
Types of Data Analytics: Descriptive, Predictive & Prescriptive Data Analytics
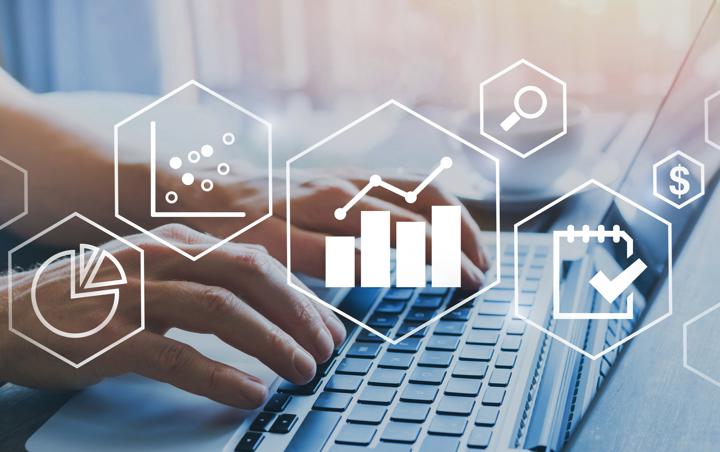
Descriptive analytics refers to the preliminary stage of data processing in which a summary of historical data is created in small, easily understandable nuggets of information, which can then be used for further predictive and prescriptive analytics. It is thus the simplest form of analysis and does not try to establish any cause and effect relationships. Data aggregation and data mining are the two processes used to report historical events in descriptive analytics. It answers the question: “What has happened?” Examples of descriptive analysis could be reports about a company’s historical sales figures.
Predictive analytics tries to answer the question: “What might happen?” It provides estimates about the likelihood of a future outcome and can be used for forecasting sales or customer behavior patterns. Predictive analytics helps you understand future possibilities, based on probabilities. The processes used are statistical models and algorithms to capture relationships between various data sets. A simple and very common example of predictive analysis is in the e-commerce sector; when you buy a product online, the interface will recommend other items for purchase based on what others have also bought.
Prescriptive Analytics is also referred to popularly as the “final frontier” of data analysis and provides advice on possible outcomes or provides guidance towards a solution before a decision is made. This type of analysis considers information about past and current performance, possible situations, and available decision options to prescribe possible actions. Prescriptive answers not just “what can happen?” but also “when it will happen?” and “why it will happen?” The processes used are a complex mix of data modeling, algorithms, and ML. If implemented accurately, prescriptive analytics can make a hugely positive impact on business decisions.
Data Analytics and the Bottom Line
The popular adage “what isn’t measured can’t be managed” has now become a veritable mantra for top management leaders. Most teams acknowledge that using data in decision-making leads to better outcomes, as decisions can be based on insights rather than leadership instincts. Mature data analytics frameworks enable organizations to take actions that hit the business bottom line and also prepare the organization to further harness AI and predictive and/or prescriptive analytics, which in turn drive further positive and impactful results.
However, in the past few years, the exponential increase of data has left many organizations in a conundrum, with poor quality of data, fragmented sources of data, lack of the right experts to find meaningful insights and recommend actions.
So, what are some ideas organizations can implement in order to use data analytics capabilities to decrease costs and increase revenues?
- Keep a regular check on the health of the organization by studying data and identifying where cost efficiencies can be improved and how much scope there is to increase revenues in various areas
- Bring in the right experts. While data science is a new and emerging science, many skilled resources exist who can help you analyze data and derive insights from your business data. Implementing data analysis technologies using the right people is key to deriving a beneficial impact from data.
- Use customer-centric data to tailor your products or services to the needs of your target audience and thereby deliver a better experience to them.
- You can also segment your target audience based on customer data and create highly personalized options at scale. Such targeted personalization can significantly drive up customer engagement.
- Developing a good, stable relationship with customers also helps win repeat business from existing customers and enhances the Customer Lifetime Value (CLV) of the customer to your business.
- Improvements in customer satisfaction and retention can boost revenues and improve profit margins.
Organizations need to work out operating models, bringing together the optimal combination of data science expertise and cutting-edge technologies to make that vital connection between using data analytics and business bottom-lines.
In conclusion, the modern enterprise must develop the capability to collect, process and standardize its business data and further transform the data into useful information that can provide actionable insights to make powerful business decisions. In order to remain competitive in today’s business landscape, organizations must ensure the following:
- Decisions that affect the business bottom lines must be data-driven
- Historical data must be leveraged to identify trends
- Data must be used to set realistic goals and KPIs (key performance indicators)
- Customer-centric data must be used to innovate and improve product or service levels
- A data-driven culture must be nurtured in every aspect of the business to strengthen the data analytics framework of the organization
Going forward, it is the organization that can extract valuable insights from data, leveraging analytics as well as the power of AI/ML, that will gain an advantage over traditionally-run businesses. Most importantly, this is now true across the entire spectrum of industries and is not limited to just technology companies.
So, are you ready to move into an era powered by data analytics and smart algorithms?