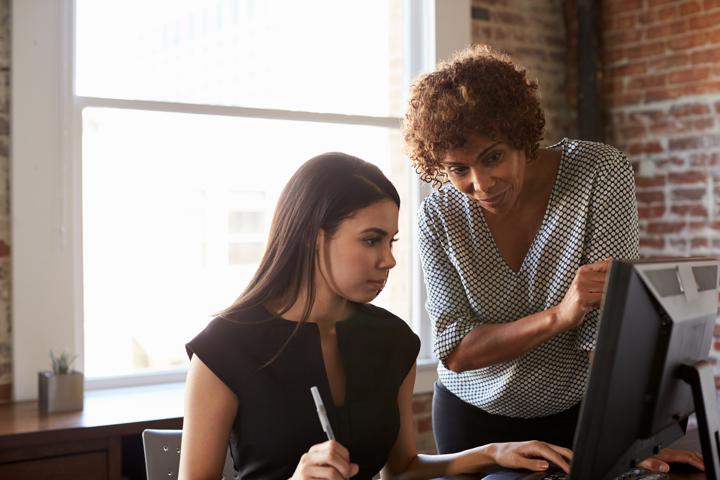
The modern enterprise deals with a staggering amount of data, which is mostly unstructured and comes from a diverse number of sources both inside and outside of the organization.
Organizations are realizing the importance of gathering, processing and storing this vast amount of business data in order to derive actionable insights and improve the organization’s performance at multiple levels.
This data is not just massive in terms of volume, but also in its complexity. Just how big do we mean? Depending on the size of the organization and the spread of its business activities, this data could well go into not just terabytes, but now counting into petabytes and rapidly moving towards the newest denomination, which is exabytes of data! And that is why it’s called ‘big data’!
Clearly, this amount of data needs advanced analytics tools to process, interpret and make sense of it. These tools must go beyond the traditional analytics tools and techniques, not just in their scope, but also in their accuracy and the scale of data they can handle. Accordingly, the data analytics industry is making giant strides towards advanced analytics and the tools are becoming easier to use.
The scope of data analytics has now moved beyond mere analysis of structured data and visualization of that data to predictive models of data and analytical processes that deal with a vast amount of semi-structured as well as unstructured data. These are the tools and techniques of next-generation data analytics, involving machine learning strategies, platforms such as Hadoop and other visionary techniques. However, most enterprises today are still stuck in the rut of business intelligence tools, custom reporting and data dashboards, and have not yet implemented the more advanced forms of data analytics, much less used their predictive powers.
As organizations begin their journey towards digital transformation and begin to process and analyze big data, there are certain agreed-upon best practices that data scientists are advocating. Let’s take a look at some of these:
1. Identify and focus on clear objectives:
As we have seen, big data is a trending topic in the industry, making it susceptible to superficial attention. Many corporate leaders become so preoccupied with simply adopting the latest technology or trend that they fail to identify clear business outcomes as the objective of the adoption exercise. This predisposes the implementation teams to failure in terms of achieving any significant business gains from the implementation.
It is crucial for organizations taking up advanced analytics of big data to focus on a specific business challenge or problem and target its resolution using data-driven insights. Clarity on what data needs to be collected, what needs to be sent for further processing and which analytics techniques and tools need to be used is essential; all of these need to be strategically defined and articulated in order to avoid wasting time and resources on data that may not deliver any actionable insights to improve performance.
2. Prepare infrastructure and technology:
Multiple tools and technology platforms are needed to support new initiatives in big data analytics. Legacy information systems are not geared toward handling big data. The existing data systems may need to be re-engineered and disparate data sources and formats may need to go through a data preparation stage first. Data storage methods may also need to be reexamined to determine whether such a vast amount of data is required to be on cloud or on-premise, and what kind of computing power may be required to deal with the processing of that data. Newer data capture methods, such as beacons, apps or sensors, will come into play as big data becomes more pervasive. A large number of software and tools are available in the market to help pre-prepare data for advanced analytics. Investing in these tools may be key to delivering results in the implementation of big data analytics projects.
3. Follow an iterative approach:
By its very nature, big data is dynamic, complex, and time-sensitive. Therefore, tools designed to analyze this data need to be agile. When processing big data, it is best to take small steps and make further iterations to accommodate new scenarios, changes in data patterns and variations in live data. It becomes very important for advanced data analytics projects to be flexible and adapt their processes — and maybe even the scope of the project — to changes in scenarios. An iterative approach allows data scientists and analysts to validate data requirements at every stage of the project and ask new questions as data changes. This allows modifications to be made in an agile manner, saving both time and cost. Dynamic data also needs to be monitored continuously and analytics processes need to be periodically reviewed and reconsidered to make sure that they are still valid in the face of changes to data.
4. Create Centers of Excellence (CoEs):
Establishing a Center of Excellence for big data analytics in your organization will imply having highly skilled experts, delivering cutting-edge analytics work and following best-in-class industry practices. The CoE thus ensures that a combination of the highest quality of people, processes and infrastructure is in place. The CoE also places emphasis on training analysts, as the best and most modern tools are ineffective unless used judiciously. The CoE also enables the transfer of knowledge in a systematic and structured manner. It values data as a strategic asset of the organization and usually partners with the business leaders to prioritize certain data projects that are of strategic significance to the organization’s goals and business targets. Last but not the least, the CoE can optimize performance, drastically cutting time-to-market for data-driven products or services and shortening implementation time significantly.
5. Build a culture of data:
Adoption of advanced analytics is a transformational initiative and requires a change of mindset through the ranks and files of the organization. It cannot and must not be seen as an ‘IT’ initiative relegated solely to the IT team. Rather, it requires C-Suite focus and a commitment towards change management. In other words, care must be taken to make it an enterprise-wide initiative and a buy-in must be created from employees across different functional areas and departments. Data collection, processing, and storage tools and platforms should be chosen with the awareness that employees at all levels will need to be able to fit the new processes into their workflows. Accordingly, they must be able to see the benefit in following newer processes or modified workflows. Data literacy throughout the enterprise, and a resultant data-driven culture, will go a long way in helping the enterprise derive rich insights, thereby making incremental performance gains as the analytics program and structure becomes more mature.
Challenges to big data analytics:
Though big data analytics is still in its infancy, data scientists and business leaders have reached some consensus on the best practices that organizations should follow in order to drive successful implementation of big data analytics tools and platforms. The possibilities are exciting and there is a lot more to learn, implement and achieve.
At the same time, the advanced analytics industry is facing some big challenges, such as finding qualified, skilled and experienced data scientists and analysts. Filling skill gaps in advanced analytics is a tough task for any CTO. Since the industry is still in infancy, previous experience is limited, making creativity and problem-solving abilities crucial, yet difficult, skills to find.
A related challenge is providing technology infrastructure at scale. With vast amounts of data being processed, organizations need more computing power, better storage options, and faster processors to deal with real-time data. Technology providers need to stay on their toes to meet the increasing demand for IT infrastructure geared towards big data analytics.
Lastly, the perennial challenge for technology companies has always been data privacy and security. Whenever large amounts of data need to be collected, stored and processed, the issue of data security comes to the forefront. Organizations that handle customer data or third-party data need to have stringent regulations in place to avoid data misuse or theft.
In conclusion:
Given the challenges that the big data analytics industry is facing and given the exponential increase in big data expected in the near future, data scientists and analysts certainly have their work cut out for them! Scale, scope and volume are all essential aspects of big data, and enterprises will need to develop expertise in handling these if they are to remain competitive in a digitalized world.
Software and technology providers also need to come up with innovative, easy-to-use tools to counter the complexities of adopting advanced analytics. As Bill Abbott, Principal for PwC recommends, “Organizations must adopt a defined analytics strategy, focusing on the repeatability of valuable analytical processes, improving productivity levels in gathering and processing information.”